Conformal Prediction Key Ingredient Enabling Safe AI: Application to Medical Coding
Repo Under Active Development
This project explores the use of conformal prediction to safely deploy AI tools when a Large Language Models (LLMs) is used to approximate medical coding. The goal is to create a system that can be used to assist medical coders in their work, without replacing them. The system uses large language models to generate suggestions for codes. Performance of LLMs at this task in the literature is highly variable and may not be suited to a particular risk profile and application.
A black-box model (such as that provided by openAI) comes with no valid assumptions. No assurances can be deduced from “how the model is” constituted, having no premises to start from. Hence, one resorts to empirical observation, “what the model does”. Such black-box systems (perhaps also “hairless” is appropriate) are very common, and the only remedy is a protocol that is extremely familiar to clinical pathologists–assay validation (in this case, measurement process is a digital computation and the measurand is per-sample prediction model reliability.
More formally, Conformal Prediction is a set of procedures and analyses which are suited to this purpose exactly. In the most straight-forward configuration, after model training is completed, the weights are frozen and a hold-out data split is used to generate iid model predictions (which are confounded by the model learned parameters on the training set) to create a statistical model of the per-sample uncertainty in the LLM’s prediction. In this project we rely on counting occurances of discrete output classifications (rather than natural language readouts) to make the statistics tractable.
Part and parcel of this procedure is a per-sample measure of “prediction difficulty”, which even if noisy and approximate, the confidence heuristic (called the nonconformity score) is tremendously useful. Predicting model performance allows a triage system where preferentially easier predictions are automated. I like to refer to such setups as ‘Graded Automation’. Concretely, the coder can choose to accept or reject the suggestions based on their confidence level. On a more abstract level, the degree of automation is itself is now being automated. The inner automation is difficult to predict and poorly understood because a LLM is used to as the model. The outer automation concerns setting a threshold, which has few degrees of freedom which makes tractable deriving statisical constraints on future performance. (any perfomance change in deployment will be akin to the degree of overfitting of a 1-Dimensional linear classification model.
In contrast with existing paradigms which rely on hand-crafted, bespoke protocols appropriate to each clinical situation. Notwithstanding human error limited clairvoyance, a method that is completely organic and unstructured cannot be improved on. By contrast, in this work the rules scoping the AI automation can be safely and automatically determined, allowing the model to abstain on the most difficult examples. Increasingly lower difficulty samples are futher witheld (possibly witholding all examples) until the model performance a predetermined performance specification can be gauranteed. This allows for a more flexible and robust system, which can adapt automatically overtime to additional use-cases as the model improves (again, with certifiable analytic validity).
Related Talks and Posters
API 2024
(poster session, Ann Arbor, MI, 2024)
Conformal Prediction and Large Language Models for Medical Coding
ACLPS 2024
(oral presentation, Ann Arbor, MI)
Graded Automation:
Using Conformal Prediction to Safely Deploy AI Tools in Medical Coding
ACLPS 2024 Young Investigator Award</br>
We are proud to announce that our work on "Conformal Prediction and Large Language Models for Medical Coding" has been recognized with the ACLPS 2024 Young Investigator Award. This award is a testament to the innovative approach and potential impact of our research in the field of medical coding and AI.
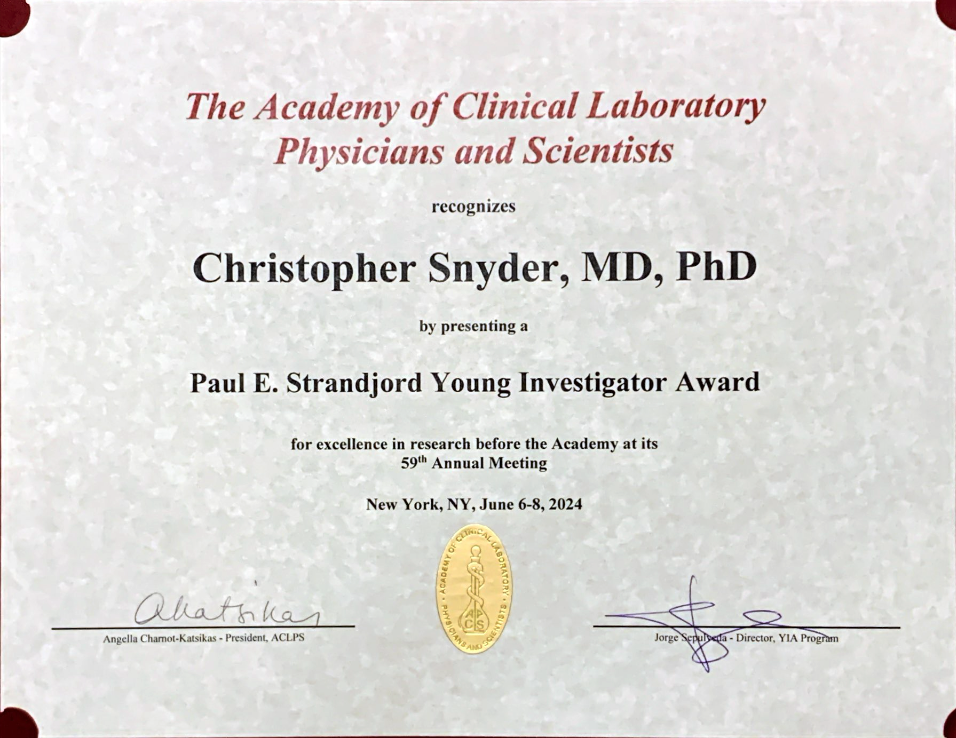